What Semantic Search Can Do for You
In the near future, will we interact with data using only natural language?
05.03.21
Search permeates our lives. Maybe we’re trying again to find the apartment keys, or perhaps it’s about searching for an affordable flight on the web — search is and will always be present. Especially in our digital lives, it is difficult to deal with the ever-increasing volumes of information. What we’re looking for is often hidden deep inside all sorts of poorly organized text data. It becomes even more challenging within an enterprise environment where looking for an important piece of information feels more and more like searching for a needle in a haystack.
Semantic Search and Search Engines
Search engines work by crawling the web or a local document database. They then output the information deemed most relevant to a given query, based on many proprietary ranking factors. The most basic search method is keyword-based. It returns the search results with the highest lexical overlap with the query. Over the years, popular web search engines like Google and Bing have been pushing for a more semantic search engine that could better understand user intent. Understanding the intent behind a search query goes a long way towards providing more relevant search results.
One way to provide search engines easier access to the contextual meaning of information is by structuring the data in a knowledge graph. Knowledge graphs help search engines to better recognize user intent and context by understanding how the entities in a database are related to one another.
Semantic search solutions, such as knowledge graphs and natural language processing (NLP) techniques, have allowed search engines to handle unstructured textual data and match documents to queries based on semantics rather than lexical overlap. A hybrid search engine can use keyword-based search alongside knowledge graphs and semantic search to get the best of all worlds.
Should We Settle for a Keyword Search?
Search tools, often based on simple keywords, are integrated into almost all modern applications. These search tools have saved us much time and effort to find information in our often overwhelming enterprise databases, wikis, websites, or applications. However, in many cases, they still fail. Instead of providing a particular piece of knowledge or pointing in the right direction, these traditional tools fail to meet the searcher's intent.
Lately, modern technologies such as Natural Language Processing (NLP) have enabled a new paradigm of search and offer the power to massively improve our search experience. In short, NLP will improve search accuracy. In particular, NLP allows us to shift, where applicable, from elaborate keyword-based matching towards the semantic understanding of our queries and questions. This way, we do not necessarily have to remember specific keywords or terminology to retrieve the desired information. Semantic search technologies such as Question Answering (QA) enable us to query text data by simply typing our questions into the search bar, then getting full answers rather than just a bunch of links to the documents matching the keywords.
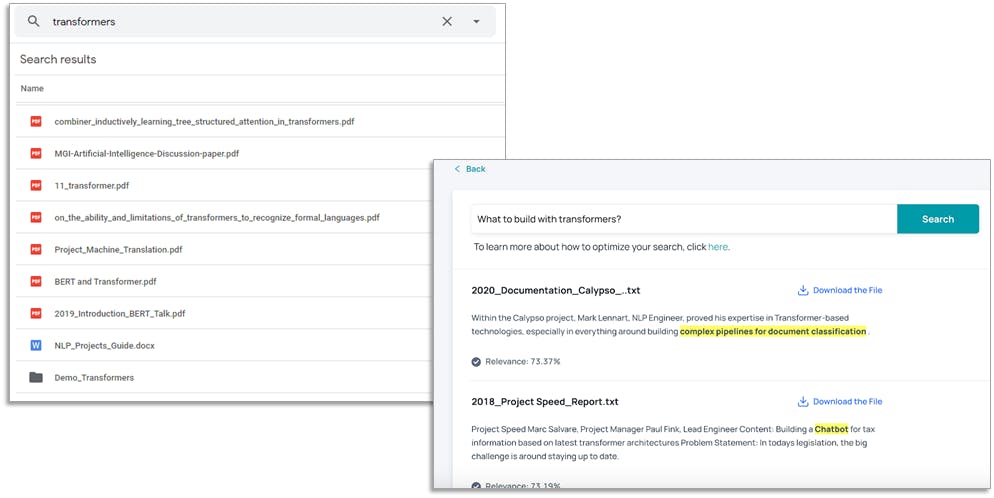
However, when does semantic search enhance our search experience, and when would a keyword-based search be sufficient?
Navigational vs. Informational Search
Based on the learnings from our clients and the open source community, we’d argue it all depends on the search intent. Almost every search query is triggered by an intent. We do not search because searching is so much fun. We search because we aim to meet a specific objective. For example, we may need to find a relevant website, explore an interesting topic, come up with an important decision, purchase a much-needed item, or maybe we’re just learning a new language. These intentions make the search either informational or navigational by nature.
Navigational search queries are driven by the need to be directed to a certain point on the Internet or inside the enterprise database. We use navigational search when we have a general idea about what we need to find, but we do not know a direct path and use the “navigation” to suggest one place after another.
This means when conducting a navigational search within enterprise databases our intent is similar to checking “points of interest” in a car navigation system or perhaps entering an incomplete address step-by-step by following suggestions.
In some cases, we also use navigational search to browse for a collection of items. For example, when searching for “project management tools,” we aim to investigate various applications to identify and select the one that fits best. We have an idea about the “destination point,” and we’d like the search engine to suggest various options to pick from.
In many cases, keywords are still sufficient for navigational search.
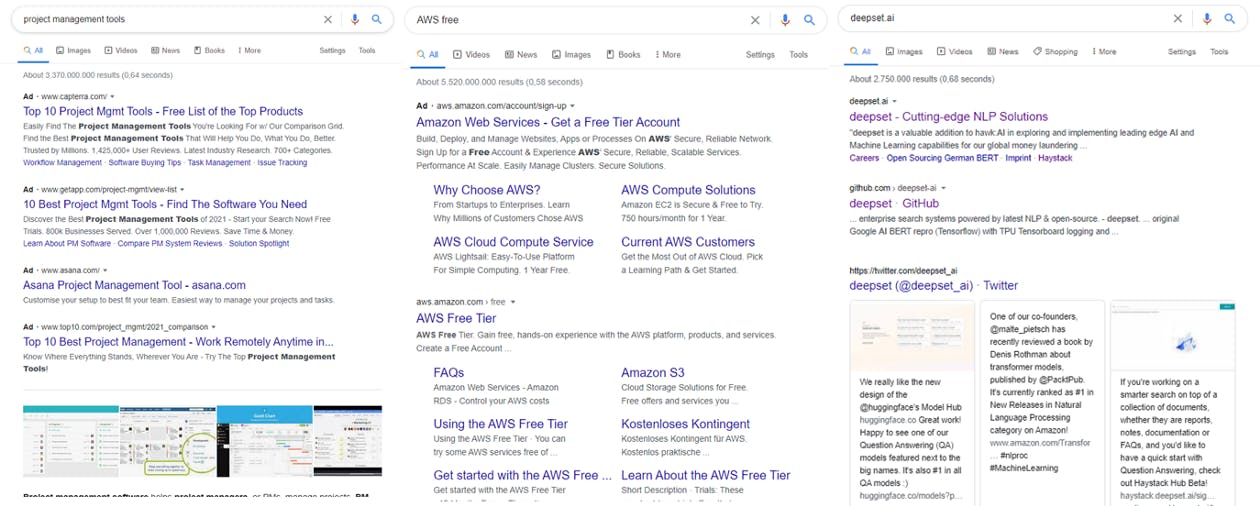
Informational search is something different. It is usually about the intent of accessing the knowledge directly and right away — it’s not about browsing a collection of potentially relevant results from a keyword-based search. Informational search is also usually stemming from having a certain question in mind.
We often do not know where to look for that particular result. We may be lacking specific terminology to describe the desirable answer, especially when being a newcomer to the knowledge area. Because we don’t know in advance what “keywords” the answer will contain, we can’t quite rely on a keyword-based search in this situation.
Our experience of helping enterprise customers with the above suggests that a semantic search can overcome the limits of a keyword-based approach and drastically improve informational search results.
What Semantic Search Can Do for You
The creation of and interaction with the knowledge is a major part of an enterprise environment. Many efforts have been made to preserve the knowledge by accumulating reports, executive summaries, meeting protocols, and other unstructured data. But as organizations grow, the amount of such data grows beyond being manageable. Finding a piece of information becomes much less feasible unless we put search terms into context.
Think maybe of someone who wants to learn about the applications for Transformer-based NLP models and what they have been used for within the company. It is unlikely that putting together a search query containing just the keywords “transformer,” “applications,” and “built” will lead to meaningful information — the context has been lost here.
In turn, semantic search allows us to directly leverage that specific question that we wanted to “apply” against the text data, for example, by asking, “What to build with transformers?”
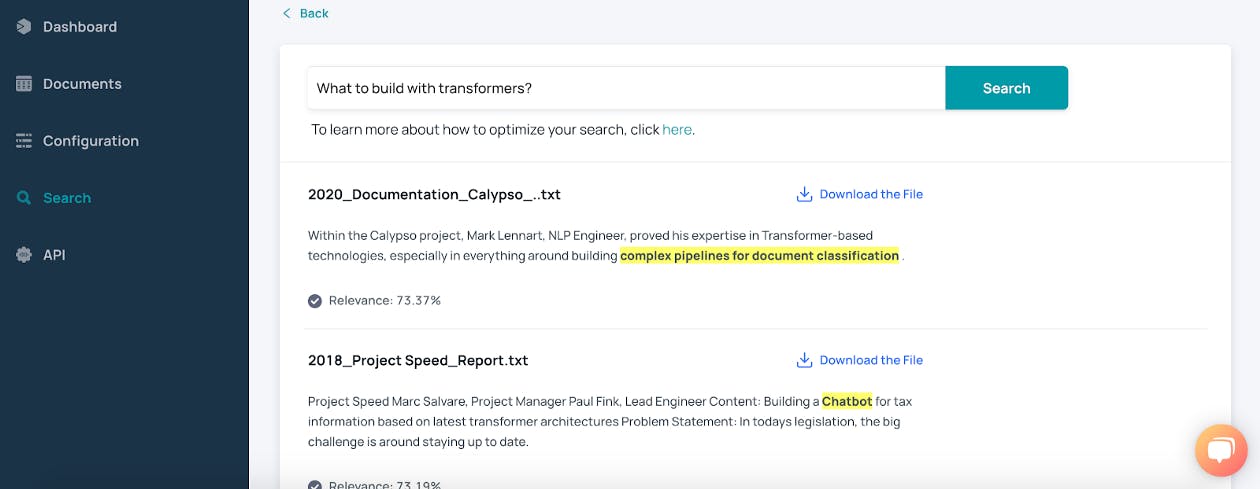
However, knowledge in the enterprise context also goes beyond just quickly solving yet another 80/20 rule and pinpointing those twenty percent of documents containing the most relevant answers. Managing the knowledge within the enterprise is also about finding people — for instance, the experts in a certain field.
The vast amount of documentation about previous projects often speaks of not just what has been done but also about who has done it. With a semantic search engine, it is unnecessary to read the reports one-by-one to identify people with specific expertise. The search will tell us exactly just who’s got the experience we are interested in.
To illustrate this, in the example below, we are looking for someone “Who has experience with Transformers?”
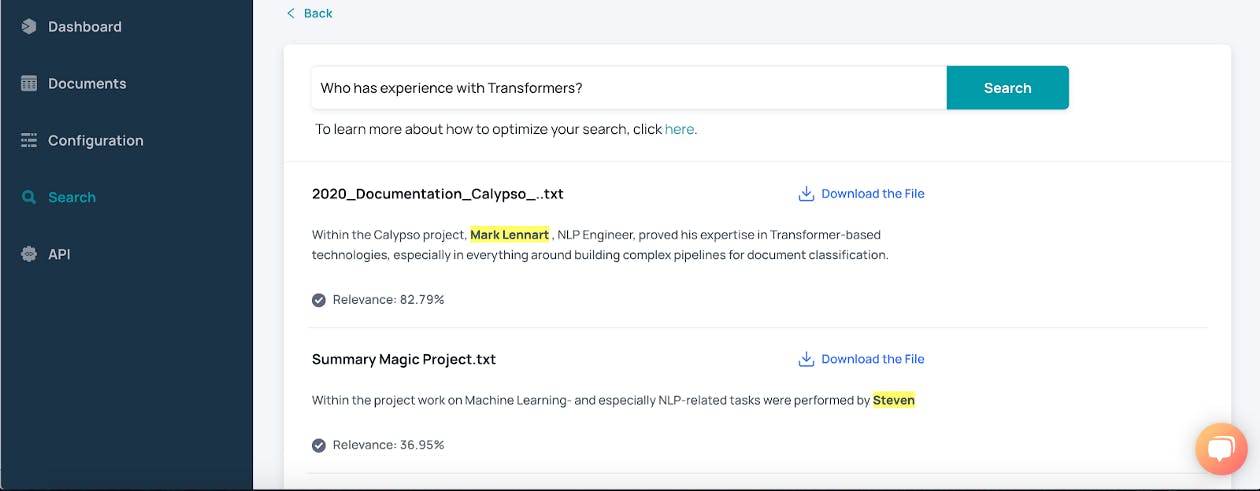
Natural language processing enables search tools with a semantic understanding of text data and the queries. They, therefore, serve as a natural language interface to interact with enterprise knowledge. These semantic search engines will no longer offer just a simple “keyword matcher.”
Instead, they provide an interface to interact with information in all formats bringing us closer to much more generic and flexible virtual assistants. One particular driver for this kind of search will also be the pervasiveness of conversational AI — chatbots and voice assistants.
We believe all of the above will lead further away from using rigid keywords and will push the search systems to interpret the most powerful communication protocol — human language.
Semantic Search on the Web
Semantic search makes it easier for you to find what you are looking for. Rather than trying to find the keyword combination that will best meet your search intent, semantic search allows you to phrase questions in a natural manner, even when you don’t exactly know what you are after. For example, if you are thinking of an old movie but cannot remember anything about it other than it was based on some board game, you can search Google for “what’s the movie about the board game.” It will return top results for several movies based on games such as Jumanji, Zathura, Ouija, Battleship, Clue, etc. The next top result is a link to a list of movies based on board games. In addition, Google uses semantic search to gauge user intent and provide factual answers directly on the search page, via the Google Knowledge Graph or featured snippets that show film posters. So not only do they link to sites that might have an answer elsewhere, they try to provide an answer directly to you by inferring what you meant. In a keyword-based search, the results would likely just include links about board games or movies. A semantic search, with a Transformer-based model, is more intuitive and conversational between the user and search engine.
On YouTube, searching for “the price of fish,” if that’s all you can remember about some song from the 90’s, returns a documentary of the same name as well as Scooter’s “How Much Is The Fish?” music video. Here, YouTube clearly recognizes the meaning and variations of a word such as “price.” Additionally, YouTube also uses search history and subscriptions, like if you subscribe to channels that feature 90’s music videos, to evaluate the relevance of what results it should provide. New machine learning-driven models, like the Transformer-based methods used by Google and YouTube, create representations of text that are semantically meaningful, giving web or enterprise search engines an understanding of language closer to how people actually use it. Ultimately, this creates a closer connection between you and all of the information at your disposal.
Enter Haystack
Over the last couple of years, we’ve developed Haystack — our open source NLP framework to build end-to-end question answering systems and semantic search pipelines.
Haystack offers the most practical way of implementing information-intensive search workflows by providing a natural language interface for unstructured data. It’s aimed at developers who just need the job done while bridging the gap between the most recent achievements in NLP and the real-world user requirements.
We want to thank everyone who’s interested in Haystack and has been supporting us through our journey. We’d encourage you to join our friendly community, and please help us spread the word by starring the repository and following us on Twitter.
Stay tuned for more insights around Haystack!