Open Sourcing German BERT Model
Learn insights into improving the original BERT model accuracy for the German language
German BERT Model
We are proud to release our first non-English BERT model — German BERT. It allows the developers working with text data in German to be more efficient with their natural language processing (NLP) tasks. German BERT model outperforms Google's original BERT model and enables new ways of instrumenting non-English question answering systems.
Berlin (June 14, 2019) — Today we are excited to open source our state-of-the-art German BERT model trained from scratch. German BERT model significantly outperforms Google's multilingual BERT model on all 5 downstream NLP tasks we've evaluated.
In this post we compare the performance of our German model against the multilingual model and share the insights we gained along the way.
Updated October 2020: We published a new generation of German Language Models that outperform GermanBERT. Check out the COLING Paper "German's Next Language Model": https://www.aclweb.org/anthology/2020.coling-main.598/
Updated July 2020: If you want to deploy and scale BERT models for neural search and question answering, check out Haystack — our flagship open source framework to build solution-centric NLP backend applications.
Updated June 2020: We have just released two new datasets, three models and a paper to push forward German natural language processing. You may also want to read our blog article on GermanQuAD and GermanDPR here.
Updated July 2019: We also released our new transfer learning framework — FARM. Check it out for a simple one-click evaluation and adaptation of German BERT: https://github.com/deepset-ai/FARM

The original BERT (Bidirectional Encoder Representations from Transformers) model was open sourced by Google in late 2018. That allowed many other NLP researchers and enthusiasts in the world to train their own state-of-the-art question answering systems. Unlike various older models that BERT built upon, BERT became the first deeply bidirectional, unsupervised language representation, pre-trained on Wikipedia. Pre-trained models are almost off-the-shelf — they can be plugged into an existing application to make it language-aware by leveraging the latest trends in natural language processing. Developers can also fine-tune models like BERT to their specific tasks by leveraging much fewer labels as compared to training from scratch.
BERT is a variant of a Transformer model — it applies the bidirectional training of Transformers to language modeling. As a result, BERT became instrumental in boosting recent research and practical applications of a variety of natural language processing tasks, such as question answering, semantic search, text generation, summarization, and others.
Why German BERT Model?
The team at deepset is committed to showing that natural language processing does not have to imply English NLP. We wanted to streamline the process of implementation of scalable neural search and question answering systems for our users in Germany, and we also wanted to make it all open source.
Although the multilingual models released by Google have increased the vocab sizes (> 100k tokens) and covered quite a lot of German text, we realized they have limitations. Specifically, when words are chunked into smaller parts, we believe the original model will have a difficult time making sense of the individual chunks.

Details About Pre-training
- We trained using Google's Tensorflow code on a single cloud TPU v2 with standard settings.
- We trained 810k steps with a batch size of 1024 for sequence length 128 and 30k steps with sequence length 512. Training took about 9 days.
- For the training data we used the latest German Wikipedia dump (6GB of raw txt files), the OpenLegalData dump (2.4 GB), and news articles (3.6 GB).
- We cleaned the data dumps using tailored scripts and segmented sentences (with spacy v2.1). To create Tensorflow records we used the recommended sentencepiece library to create the word piece vocabulary and the tensorflow scripts to convert the text to data usable by BERT.
Evaluating Performance on Downstream Tasks
There does not seem to be any consensus in the community about when to stop pre-training or how to interpret the loss coming from BERT's self-supervision. We took the approach of BERT's original authors and evaluated the model performance on downstream tasks. For the tasks we gathered the following German datasets:
- germEval18Fine: Macro f1 score for multiclass classification — Identification of Offensive Language
- germEval18Coarse: Macro f1 score for binary classification — Identification of Offensive Language
- germEval14: Seq f1 score for NER
- CONLL03: Seq f1 score for NER (deu.train - deu.testa as dev - deu.testb as test set)
- 10kGNAD: Accuracy for document classification
Even without a thorough hyperparameter tuning, we observed quite stable deep learning — especially for our German model. Multiple restarts with different seeds produced similar results.
We further evaluated different points during the 9 days of pre-training and were astonished at how fast the model converges to the maximally reachable performance.
We ran all 5 downstream tasks on 7 different model checkpoints — taken at 0 up to 840k training steps (x-axis in figure below).
Most checkpoints are taken from the early training, where we expected most of the changes in performance. Surprisingly, even a randomly initialized BERT can be trained only on labeled downstream datasets, and reach good performance (blue line, GermEval 2018 Coarse task, 795 kB training set size).
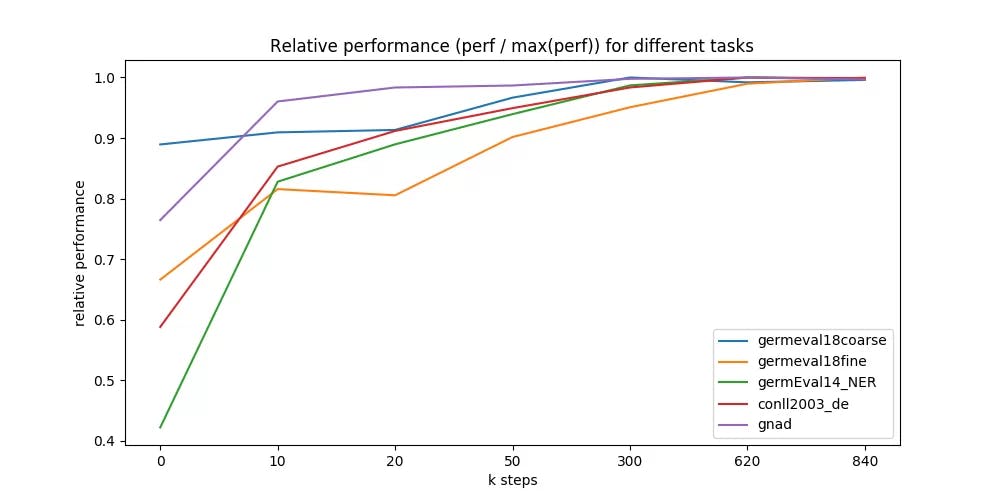
Conclusion
We hope that our work on German BERT model will help other teams to learn from this process and start making their own non-English models for natural language processing and language understanding. By using our German BERT model anyone working on implementing NLP for German language can achieve higher accuracy and better performing question answering. In the future we are planning to do more work in this area as well.
If you have any questions, want to share your insights about pre-training from scratch or using our models — feel free to contact us, and/or join our vibrant community!
Make sure to also try our Haystack NLP framework and give us a star if you like it :)